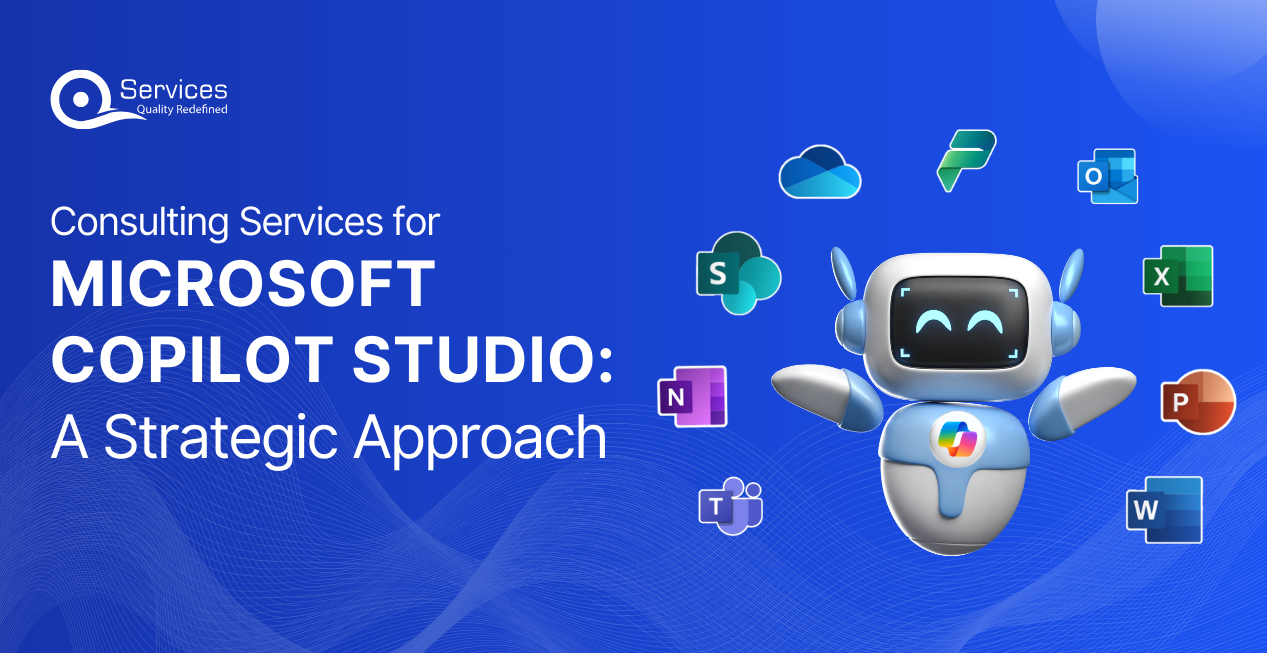
Rewards
.
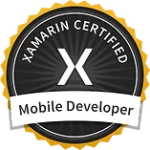
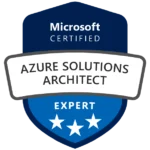
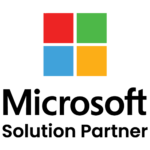
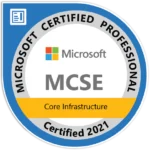
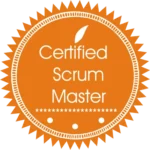
CANADA
55 Village Center Place, Suite 307 Bldg 4287,
Mississauga ON L4Z 1V9, Canada
Certified Members:
.

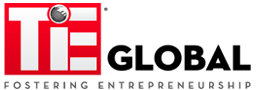
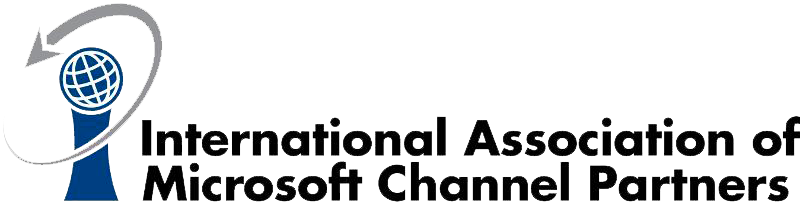
Home » Microsoft Azure Machine Learning vs Google Cloud AI Platform: A Comprehensive Comparison
Machine Learning and AI are crucial for modern businesses because they help people to make wise decisions. Microsoft Azure Machine Learning and Google Cloud AI Platform are two top choices for these technologies, making the comparison of Google AI vs. Microsoft AI particularly relevant for organizations exploring their options.
Google Cloud AI offers various AI services while Azure Machine Learning platform is great to build and deploy machine learning models. This comparison aims to help businesses decide which platform is better for their needs by looking at their features, performance, and how well they integrate with other tools. With AI expected to add huge value to the global economy, picking the right platform is very important.
Azure Machine Learning considered as a cloud-based service that streamlines the entire machine learning (ML) project lifecycle. It’s perfect for data scientists, ML engineers, and developers looking to build, train, and deploy ML models efficiently.
Azure ML services effortlessly integrate with other Microsoft services, making it a significant part of the Azure AI ecosystem. To understand it better, let’s understand how it does all that. Azure machine learning works with Azure Synapse Analytics for big data processing, Azure Arc utilizes for hybrid cloud scenarios, and Azure App Service for deploying machine-powered applications.
Compute options: You can choose the optimal resources for your machine learning applications with a reliable Microsoft AI platform like Azure ML which supports a variety of compute alternatives, including CPUs and specialist GPUs.
Automated Machine Learning: Without having in-depth knowledge of machine learning, Azure’s automated machine learning enables you to rapidly build precise models for tasks like classification, regression, and clustering.
MLOps: Azure Machine Learning services provide powerful capabilities for overseeing the whole machine learning lifecycle, which includes monitoring, deployment, and model training.
Responsible AI: The Azure AI platform has significant built-in capabilities that help make sure your models are equitable, comprehensible, and legal.
Integration with Open-Source Tools: You can use popular ML frameworks like TensorFlow, PyTorch, and scikit-learn within the Azure ML platform, making it flexible and versatile.
Get free Consultation and let us know your project idea to turn into an amazing digital product.
Whether you have expertise or are utterly naive in machine learning, the Google Cloud AI Platform has automated and custom model development tools.
A unified interface means having information in one platform, making managing everything from data prep to deploying your models easy.
Azure Machine Learning Studio is easy to use with its drag-and-drop interface, making it a breeze to build, test, and deploy machine learning models. It’s great for everyone, from beginners to pros, and it works well with other Azure services. You don’t need to be a coding expert to create complex workflows, thanks to its intuitive design and loads of helpful resources like tutorials and community support.
Google AI Platform (Vertex AI) offers a smooth, unified interface for handling the whole machine learning process. It integrates nicely with Google Cloud services and supports both automated and custom model development. You can use pre-built models or create your own with frameworks like TensorFlow and PyTorch. Plus, it has advanced features like hyperparameter tuning and model monitoring, making it easy to manage and optimize your models efficiently.
Strong features like AutoML, which streamlines the model selection and tuning process and makes it simpler for users to create and implement models, are offered by Azure Machine Learning Services. Businesses can simply handle enormous datasets thanks to its exceptional scalability and performance. This platform also smoothly interfaces with other Azure services, which improves its overall usefulness and usability.
Google Cloud Platform also offers powerful tools for machine learning, like AutoML and custom model training. It supports advanced AI capabilities and offers a range of tools for model deployment and management.
Google cloud machine learning is basically designed for flexibility and scalability as it integrates well with other Google Cloud services providing a comprehensive solution for various machine learning needs.
Microsoft Azure ML Effortlessly integrates with a variety of Microsoft services, such as Power BI and Azure DevOps which allows users to visualize data insights directly from their machine learning models and manage CI/CD pipelines efficiently.
Google Cloud AI Platform, on the other hand, integrates effectively with Google services like BigQuery and Google Kubernetes Engine (GKE). This integration supports large-scale data analytics and scalable model deployment which helps in streamlining the entire machine learning workflows. Making it a great choice for data-intensive tasks and for businesses who are constantly looking for continuous innovation.
Microsoft Azure Machine Learning Studio
Microsoft Azure Machine Learning Studio has solid security features such as network security, encryption both at rest and in transit, and identity management through Azure Active Directory, ensuring only authorized users get access. It also meets industry standards like GDPR, HIPAA, and ISO/IEC 27001, which helps businesses stay compliant with legal requirements. Plus, azure machine learning studio also focuses on data privacy with features like data masking and secure storage, protecting sensitive information from the moment it’s ingested to when the model is deployed.
Google Cloud Machine Learning
Where Google Cloud Machine Learning has security features like encryption both at rest and in transit, network security and identity and access management, protecting all sort of data from unauthorized access and threats. Additionally, Google Cloud focuses on data privacy with significant features like data anonymization and secure storage. Which ensures that data of the user is protected throughout the entire machine learning process.
When it comes to customer support, Azure shines with its dedicated account managers in higher-tier plans, offering personalized assistance for large enterprises. While on the other hand, Google Cloud excels in cost management with detailed tools and transparent pricing, helping businesses optimize their spending effectively.
In terms of community and resources, Azure integrates seamlessly with other Microsoft services, making it a great choice for businesses already in the Microsoft ecosystem.
Meanwhile, Google Cloud leverages Google’s AI and machine learning expertise, providing innovative tools and continuous advancements. For training and certification, Azure’s certifications like the Azure AI Engineer Associate are highly valued in Microsoft-centric environments, while Google Cloud’s certifications, such as the Google Cloud Professional Machine Learning Engineer, are well-regarded in the tech industry, especially for data-intensive projects.
As we’ve detailed all the significant features of both platforms, and it’s been very clear that choosing the right AI and machine learning solution is crucial for modern businesses.
So now, the choice between Google AI vs. Microsoft AI depends on your specific needs and existing infrastructure. By analysing each platform’s strengths and their alignment with your business objectives and requirements, you can surely choose the best tool for your AI projects and gain a competitive edge.
Our Articles are a precise collection of research and work done throughout our projects as well as our expert Foresight for the upcoming Changes in the IT Industry. We are a premier software and mobile application development firm, catering specifically to small and medium-sized businesses (SMBs). As a Microsoft Certified company, we offer a suite of services encompassing Software and Mobile Application Development, Microsoft Azure, Dynamics 365 CRM, and Microsoft PowerAutomate. Our team, comprising 90 skilled professionals, is dedicated to driving digital and app innovation, ensuring our clients receive top-tier, tailor-made solutions that align with their unique business needs.
Microsoft Azure Machine Learning and Google Cloud AI Platform both offer robust machine learning services, but they differ in their approach and features. Azure ML provides a comprehensive suite of tools for building, training, and deploying models, with strong integration with other Microsoft services like Azure DevOps and Power BI. Google Cloud AI Platform, on the other hand, excels in its ease of use and integration with Google’s ecosystem, including TensorFlow and BigQuery. It also offers advanced AI services like AutoML for automated model training.
Both platforms offer pay-as-you-go pricing models, but the specifics can vary. Azure Machine Learning charges based on compute resources, storage, and specific services used. Google Cloud AI Platform also charges based on compute and storage, but it often provides more granular pricing options for specific AI services. It’s important to review the pricing calculators and documentation of both platforms to understand the costs for your specific use case.
Common use cases for both platforms include predictive analytics, natural language processing, image and video analysis, and recommendation systems. Azure Machine Learning is often used in industries like finance, healthcare, and manufacturing for tasks such as fraud detection, predictive maintenance, and personalized medicine. Google Cloud AI Platform is popular in retail, media, and technology sectors for applications like customer segmentation, content recommendation, and automated customer support.
Both platforms offer features to facilitate collaboration. Azure Machine Learning provides workspaces, version control, and integration with GitHub and Azure DevOps. Google Cloud AI Platform supports collaboration through AI Platform Notebooks, shared datasets, and integration with GitHub and Cloud Source Repositories.
Azure Machine Learning offers extensive customization options, including custom machine learning pipelines and support for various programming languages. Google Cloud AI Platform provides flexibility through custom model training and integration with multiple AI frameworks.
Azure Machine Learning supports Python, R, and various machine learning frameworks like TensorFlow, PyTorch, and Scikit-learn. Google Cloud AI Platform primarily supports Python and TensorFlow but also integrates with other frameworks like PyTorch and Scikit-learn.
Azure Machine Learning integrates with Azure Synapse Analytics and Azure Data Lake for large-scale data processing. Google Cloud AI Platform leverages BigQuery and Dataflow for handling big data analytics.
Both platforms offer robust disaster recovery options. Azure Machine Learning provides geo-redundant storage and backup solutions. Google Cloud AI Platform offers multi-region storage and automated backups.
Azure Machine Learning supports edge computing through Azure IoT Edge and Azure Stack. Google Cloud AI Platform integrates with Google Cloud IoT Core and Edge TPU for edge computing solutions.
Azure Machine Learning supports deployment to Azure Kubernetes Service (AKS), Azure Container Instances (ACI), and Azure Functions. Google Cloud AI Platform offers deployment to AI Platform Prediction, Kubernetes Engine, and Cloud Functions.
Schedule a Customized Consultation. Shape Your Azure Roadmap with Expert Guidance and Strategies Tailored to Your Business Needs.
.
55 Village Center Place, Suite 307 Bldg 4287,
Mississauga ON L4Z 1V9, Canada
.
Founder and CEO
Chief Sales Officer